Machine learning answers the question what is a business target (sales, number of customers, churn of customers) going to be from past data on inputs and business targets. The machine learning algorithms find very exactly any associations between inputs and business targets. We get very precise and complex functions, mapping inputs to business targets. However, these types of questions are only a small part of business decision making. A large part of business decision making are what if questions. What if we increase one of the inputs e.g. spent more on marketing, are we going to improve on important business targets like higher sales from existing customers or attracting more new customers. To those questions machine learning is typically not the right answer. Following illustration of why data science needs both machine learning and causal data analysis. Your goal is to create a personalized advertisement algorithm to better target your customers. You begin collecting past data about product sales, marketing efforts and your customer base. Based on this data you train a machine learning algorithm that sends personalized advertisements to each customer, which should maximize the sales. After developing, you show your results to your business colleagues. They are somewhat skeptical and challenge you to show that your approach outperforms their business rules.
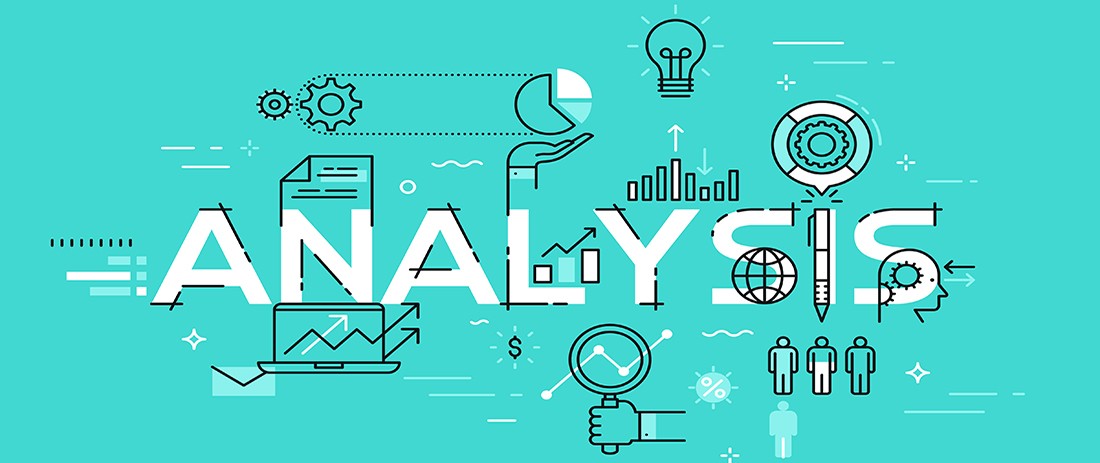
Causal data analysis offers a solution to test the performance of the two approaches against each other. You set up a random assignment of customers to a treatment group, which receives the personalized advertisement, or into a control group, which is targeted according to the old business rules. After comparing the sales of those two before and after introducing the new personalization algorithm in the treatment group, you can confidently point out to business the benefits of your algorithm.
But which techniques can data science use to answer what if questions for business decision making. In my illustration, I have already talked about random experiments, but many business situations experiments are either not possible or to expensive. Alternative techniques from computer science like casual graph analysis or techniques from the social sciences like quasi natural experiment approaches (instrumental variables, discontinuity design, difference-in-difference) can help in those situations to gain insights into causal effects from non-experimental data. Causal data analysis shifts one more important aspect. Not only does it answer what if questions instead of what questions, it also fosters a closer collaboration between business and data science. E.g. in a causal graph analysis a data scientist encodes a causal graph, which represents the business knowledge about the question, to estimates the effects of key inputs on targets. In quasi natural experiments, a data scientist uses sources of external variation of key inputs unrelated to the target, to understand the causal effect of key inputs on those targets. All techniques require a healthy dose of business knowledge and data knowledge.
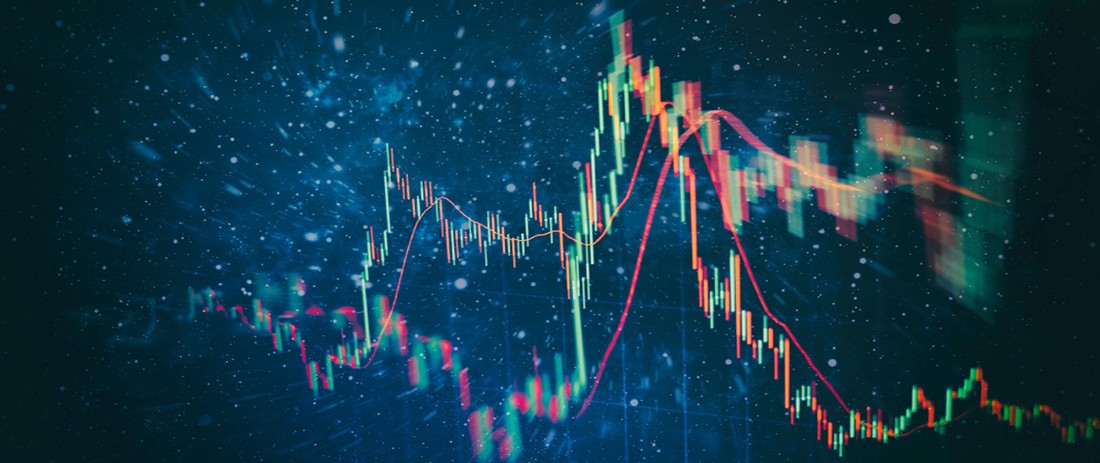
I hope that in this short article I showed you the benefit to look at causal data analysis to answer what if questions. Causal data analysis is a collection of techniques like experiments, quasi natural experiments and causal graph analysis useful to know next to machine learning to help business discussion making. Any questions? Don’t hesitate to contact marketing.belgium@keyrus.com